Understanding the AI Hallucination Problem: Examples, Causes and Prevention Strategies

AI hallucinations can result from several factors, including inadequate training data, erroneous assumptions by the model, data biases, processing mistakes, and improper use of learned patterns. These issues can pose risks for AI systems involved in critical decisions, such as medical diagnoses and financial trading.
At ServerMania, we are a cloud hosting company that understands AI hallucinations and has better ways to manage situations like this with the use of GPU dedicated server hosting or specific Nvidia GPU servers. However, we always advise against being fully dependent on AI server technology. Like every other machine, this technology can make mistakes, and these mistakes can be costly.
Using the best GPU for machine learning to store accurate training data is one way to get rid of the hallucination problem. Check out our blog on AMD vs. Nvidia GPU to get a full understanding of this technology.
Some examples of AI hallucinations include:
- Non-existing locations such as the “The Eiffel Pyramid in Rome,” which blends real concepts into a fictional entity.
- Suggesting code snippets with logical errors, invalid syntax, or referencing APIs and libraries that don’t exist.
One technique that can help mitigate AI hallucinations is retrieval augmented generation (RAG). RAG gives generative AI models access to a database that contains accurate information.
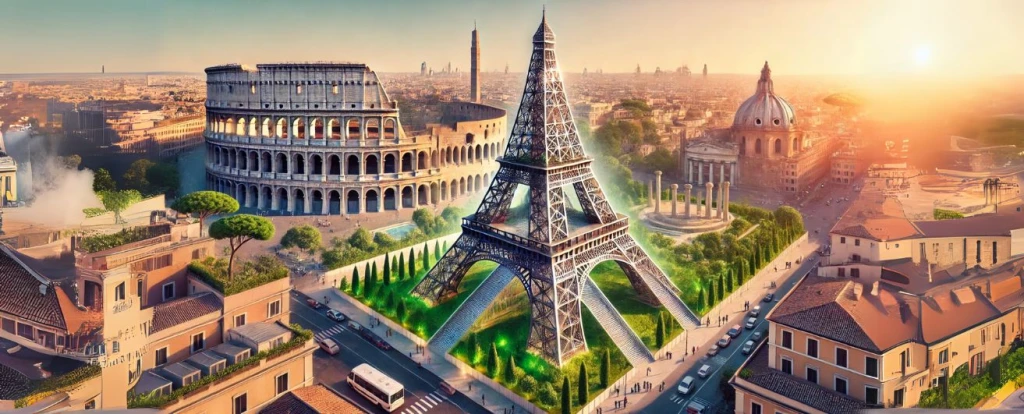
What is AI Hallucination?
An AI hallucination is when an artificial intelligence model generates incorrect or misleading results. These errors can include fabricated details, nonsensical text, responses that run against logic, and image recognition systems that see objects that aren’t there.
When using generative AI tools, users typically hope for a response that perfectly answers their request. Yet, there are times when generative AI models might produce outputs that stray from their training data or misunderstand the input, leading to unexpected results. This can result in what we call ‘hallucinations’ in the response, where the information doesn’t align with reality.
AI hallucinations occur when a generative AI model produces incorrect or misleading information, often presenting it as if it were true. This challenge can be found in all types of generative AI tools, from chatbots to language models. The hallucinations can vary from minor mistakes to serious reasoning issues. They’re typically caused by a lack of training data, biased or inadequate data, and the model’s struggle to grasp the context of a question.
When Does AI Hallucinations Occur?
This could occur when a generative AI model responds inaccurately but is statistically similar to the correct data. It can also occur when the model is trained using incomplete or biased data or if the data is misconstrued.
These hallucinations could range from minor inconsistencies to completely fabricated information. They can be challenging to detect as they often appear plausible, which can lead to incorrect decisions. For example, AI software might incorrectly state that a customer can return an item after 60 days when the actual policy is only 30 days.
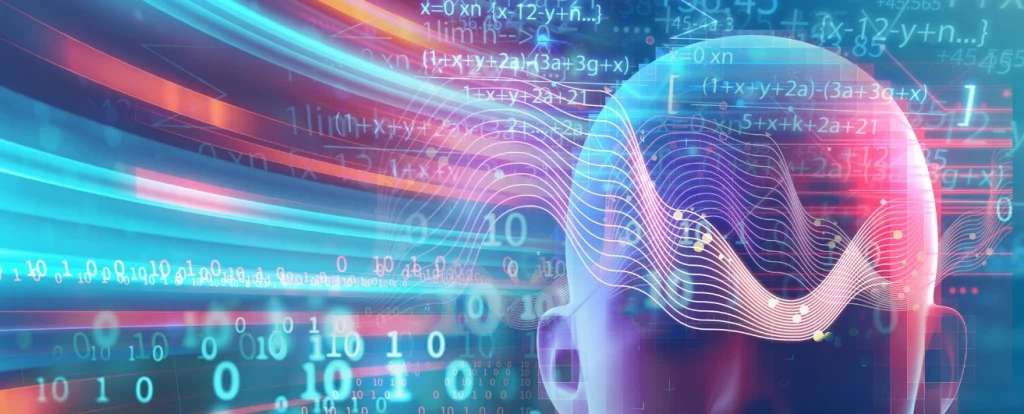
Causes of AI Hallucinations
AI hallucinations happen for a variety of reasons, such as:
- Using outdated or low-quality training data can be a real challenge. Remember, an AI model shines best when it has the right information to work with! If the AI tool struggles to grasp your prompt or lacks the necessary details, it will depend on the limited dataset it has learned from, which might lead to some inaccuracies in its response.
- Sometimes, data retrieval can be tricky! While AI technology is getting better at accessing extra data from various sources, it still struggles with confirming its accuracy. For instance, this is why Google’s AI once suggested putting glue on pizza, which is absolutely odd.
- Overfitting happens when an AI model is trained on a small dataset, leading it to memorize the inputs and their corresponding outputs. As a result, it struggles to generalize with new data, which can cause some unexpected AI hallucinations.
- When using idioms or slang expressions, it’s important to remember that if a prompt includes one that the AI model is unfamiliar with, the results might not make much sense. Let’s be cautious with our word choices to ensure clear communication!
- Adversarial attacks refer to prompts that are intentionally crafted to puzzle the AI, leading it to create what we call AI hallucinations.
- Large language models (LLMs) and large multimodal models (LMMs) don’t really know anything and can fabricate information. Their fabricated information is mostly based of insufficient training data from their developers. LLMs (Large language models) are designed to predict the best string of text that plausibly follows from a prompt without considering truth or factual accuracy.
But really, hallucinations stem from the design and training of modern generative AI models. Even with top-notch training data and clear instructions, there’s still a small chance that the generative AI model might produce a hallucination result. This issue affects all types of generative AI models, whether they’re crafting images or text.
Here, at ServerMania, we pay special attention to this model, as it tends to be the most troublesome and common form of hallucination. When DALL·E 3 doesn’t create the right image, it’s usually easy to spot. However, with ChatGPT, identifying an error might take a bit more effort.
Consequences of AI Hallucinations
- AI hallucinations can perpetuate biases or cause harmful consequences if taken at face value.
- They can also cause costly problems for companies and erode user trust.
- These hallucinations can lead to incorrect human decision-making and undermine user trust.
- AI hallucinations can sometimes create a sense of generative human speech, making it easy for users to see AI systems as having human-like qualities. It’s fascinating how we can relate to this technology in such a personal way.
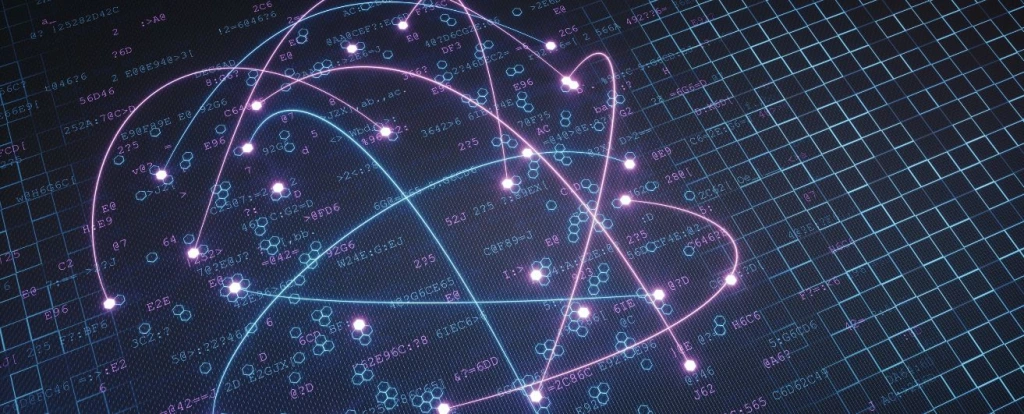
Detection and Prevention Strategies
Reducing AI hallucinations is really important to create a trustworthy and dependable artificial intelligence system.
With the use of certain strategies, we can lower the chances of these systems producing misleading or incorrect information. Here’s a simple 5-step guide on how to achieve this:
1. Use High-Quality Training Data
To effectively prevent AI hallucinations, we really need to focus on using high-quality, comprehensive training data. This means carefully curating datasets that genuinely reflect the real world, incorporating a variety of scenarios and examples to address potential edge cases. It’s important to ensure the data is free from biases and errors, as any inaccuracies can lead to undesired hallucinations. Plus, regular updates and expanded datasets are great ways to help the AI stay in tune with new information and minimize inaccuracies!
Luckily, AI hallucination is a well-known issue, and companies are working to solve it.
For instance, the newest version of Anthropic’s AI model, Claude 2.1, has made remarkable strides in accuracy, achieving a twofold reduction in the rate of incorrect statements compared to its predecessor, Claude 2.0. This exciting advancement helps businesses utilize reliable and high-performing AI solutions to tackle real-world challenges and seamlessly integrate AI into their operations.
2. Use Data Templates
Data templates act like friendly roadmaps for AI responses, helping to ensure consistency and accuracy in the content they generate. With clear outlines, a format, and an acceptable range of responses, you can gently guide AI systems away from straying into fabrication.
This approach is beneficial in applications that need a specific structure, like reporting or data entry, where everyone appreciates a standard expected output. Plus, templates play a remarkable role in reinforcing the learning process with the provision of precise and relatable examples.
3. Restrict Your Dataset
Limiting the dataset to trusted and verified sources helps the AI learn from accurate information, avoiding misleading or incorrect data. This means carefully selecting content from authoritative and credible sources while avoiding anything known to be false or merely speculative.
4. Specific Prompts
Creating prompts with precise details can really help prevent AI hallucinations. By offering specific and detailed instructions, you guide the AI to generate exactly what you’re looking for, minimizing any chances for misunderstanding.
Including context, desired details, and even citing sources allows the AI to grasp the task better and provide more precise and relevant responses. This focused approach helps keep the AI on track, steering it away from making unnecessary assumptions or creating inaccurate responses.
5. Default to human fact-checking
Even with the tremendous strides we’ve made in AI, having a human review layer continues to be one of the best ways to protect against misunderstandings. Human fact-checkers play a vital role in spotting and correcting mistakes that AI might overlook, adding an essential layer of accuracy to the system’s outputs. Regularly checking AI-generated content for any errors and refining the AI’s training data with correct information not only boosts its performance but also helps ensure that the results are reliable and ready for use or publication.
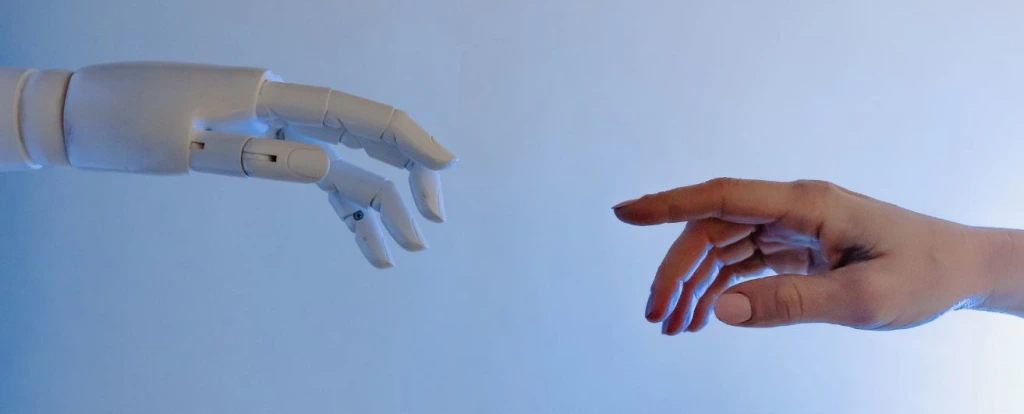
Future of AI Hallucinations
Researchers are working to mitigate AI hallucinations with better training data, improved algorithms, and the addition of fact-checking mechanisms. Human oversight is another aspect that could potentially better manage hallucinations within the scope of factual information. The EU AI Act creates a clearer and more supportive framework that fosters transparency, encourages accountability, and promotes human oversight in the development and deployment of AI.
Conclusion
Artificial intelligence hallucinations are a significant problem in AI systems, but they can be addressed with careful design and implementation. To prevent them, high-quality training data, transparent and explainable results, and detection and prevention strategies are essential.
When we understand the causes and consequences of these hallucinations, we can create generative AI tools that we can trust—systems that deliver accurate and reliable results for everyone involved!
Was this page helpful?